6G-Empowered Offloading for Realtime Applications in Multi-Access Edge Computing
6G-Empowered Offloading for Realtime Applications in Multi-Access Edge Computing
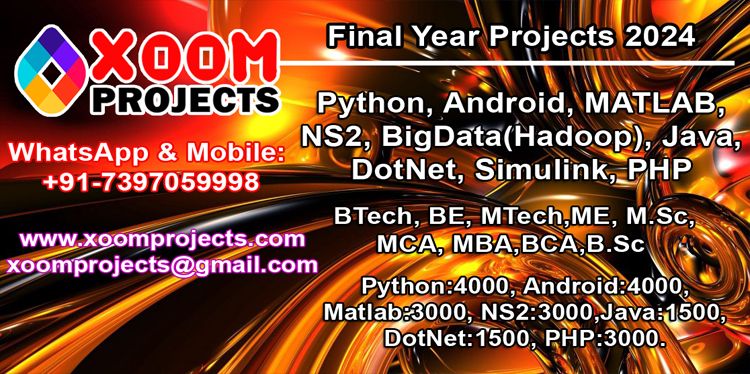
Abstract:
Multi-access Edge Computing (MEC) is a promising solution to the resource shortage problem on mobile devices. With MEC, a fraction of the computational tasks on mobile devices could be offloaded to edge servers. Over the past years, a series of machine learning based offloading methods for MEC have been proposed to reduce the completion time of computational tasks. However, most of the existing methods do not work well for realtime applications, which involve tasks with rigorous deadline constraints. In addition, offloading data-intensive tasks via the latest wireless networks, such as LTE and 5G, could lead to unsatisfactory transmission delays. Furthermore, with the state-of-the-art learning-based methods, both the training and inference operation of the learning algorithm are carried out on mobile devices, undesirably leaving less computation resources for computational tasks on mobile devices. In this paper, we propose a 6G-empowered learning-based offloading scheme, MELO, which can be used to make appropriate offloading
decisions for realtime tasks. Specifically, the task offloading problem is first formulated as a Markov Decision Process. Thereafter, the problem is solved with a Reinforcement Learning (RL) algorithm, TD3. In addition, 6G is adopted as the communication infrastructure to sufficiently support the data transfer between mobile devices and edge servers. Furthermore, to leave more resources on mobile devices, we devise a novel learning architecture, EALA. With EALA, the training and inference operation of a learning algorithm are decoupled. The training operation is carried out on edge servers while the inference operation is performed on mobile devices. Our experimental results indicate that MELO outperforms the existing offloading methods in terms of task completion time.